# 自监督视觉transformer
模型DINO
# 总体介绍
论文:1.Emerging Properties in Self-Supervised Vision Transformers (opens new window)
这篇文章旨在探索自监督训练有没有给视觉transformer
带来相对于CNN没有的新特性。
除了观测到自监督训练ViT工作特别好外,作者还有两个新发现,一个是自监督训练得到的特征图包含明显的语义信息,有可能将自监督的结果直接拿来做语义分割和目标检测,另外一个是直接拿自监督得到的特征向量应用KNN分类,得到了非常好的效果。ps:本人在工程数据(20W张)上验证的直接使用KNN分类的效果比efficient-net还好。
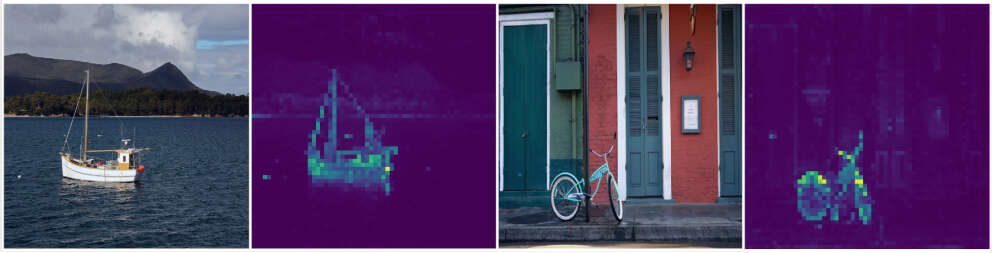
正如DINO
的名字缩写,这整个算法使用了知识蒸馏 (opens new window)的架构,通过一个teacher
网络引导student
的学习,使用损失值计算的梯度更新student
模型的参数,而teacher
模型的参数使用的是student
模型参数的指数移动平均值,和BYOL的方法有些相似。除了知识蒸馏,作者还强调了对输入进行RandomResizeCrop
和transformer
使用小patch_size
的重要性。同时,DINO
需要对teacher
的输出进行中心化和锐化centering and sharpening
,否则模型训练会不稳定,甚至崩溃(collapse)。DINO
使用的student
和teacher
且训练过程中相互促进学习,也属于共蒸馏codistillation
模型。
知识蒸馏的概念是一个学生网络student
表示为teacher
表示为teacher
引导student
的训练。
假如给定一个输入图像K
个类别的分类),student
和teacher
对应的输出概率分别为P_s
和P_t
。
在计算student
输出概率的时候使用的是带softmax
方法,在DINO
中默认的teacher
输出的概率时同样使用了
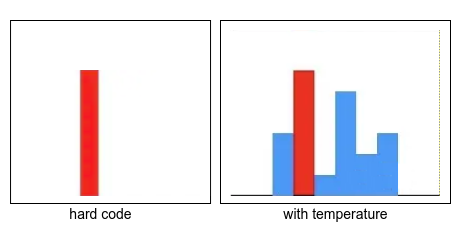
自蒸馏学习的目标函数就是最小化
# DINO中使用的SSL和KD方法
算法结构如图:
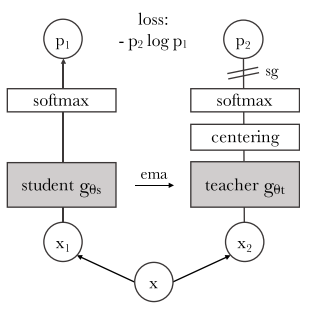
伪代码实现:
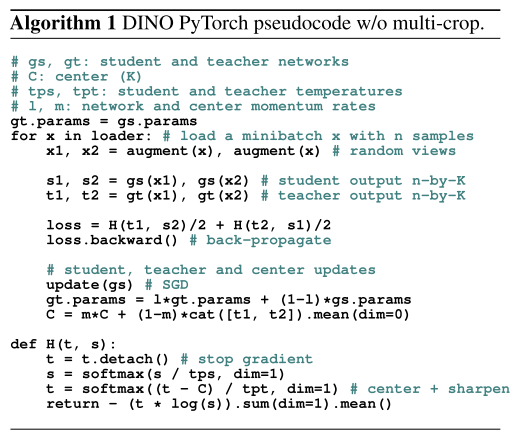
从上面两张图介绍可以看到,teacher
与student
模型结构完全相同,但与KD不太一样的时,因为是自监督训练,并没有现成的teacher
可以用,因此训练的过程中还需要更新teacher
的参数,teacher
的参数使用student
参数的指数移动均值来计算更新的。
# multicrop strategy
对于每次输入模型的数据,都会对图像进行随机裁剪,裁剪的方式分成两种:
- 全局裁剪
global crop
,每次截取输入图像上40-100%
的区域再resize
成224
大小 - 局部裁剪
local crop
,每次截取输入图像上5-40%
的区域再resize
成96
大小
# global crop
torchvision.transforms.RandomResizedCrop(224, scale=(0.4,1.0), interpolation=Image.BICUBIC),
# local crop
torchvision.transforms.RandomResizedCrop(96, scale=(0.05,0.4), interpolation=Image.BICUBIC),
这样对于输入图像,通过2
次global crop
和8
次local crop
就能得到输入图像上的多个视图。所有10
个crops
都送入student
网络计算输出2
个global crop
送入teacher
网络计算local-to-global
的匹配能力。值得注意的是,这里计算2
个global crop
与8
个local crop
对应输出之间的熵。2
次global crop
虽然送入了student
参与计算,但这里理解只是为了给student
提供上下文,辅助训练。
还有一点值得注意的是送入student
的10
个crops
大小不一致,两个224
,八个96
,其处理方式为在区分不同大小的图像,然后在batch
维度上合并后输入ViT
计算:
# x是list,每个元素的shape[batch_size, 3, image_h, image_w]
idx_crops = torch.cumsum(torch.unique_consecutive(
torch.tensor([inp.shape[-1] for inp in x]),
return_counts=True,
)[1], 0) # [224,224,96,96,96,96,96,96,96,96] -> [2,8]->[2,10]
start_idx, output = 0, torch.empty(0).to(x[0].device)
# slice(0,2),slice(2,10)
for end_idx in idx_crops:
x_in = torch.cat(x[start_idx: end_idx])
...
start_idx = end_idx
虽然对于不同大小的图像ViT
经过ImageEmbedding
后得到的序列长度不同,但是ViT
中取的是cls_token
作为输出,因此不同序列长度输出的张量维度是相同的,都是embedding_dimensions
,对于student
将不同图像的ViT
输出在batch
维度上合并即可。
output = torch.empty(0)
for end_idx in idx_crops:
x_in = torch.cat(x[start_idx: end_idx])
_out = self.backbone(x_in) # (batch_size, embedding_dims)
# accumulate outputs
output = torch.cat((output, _out))
start_idx = end_idx
# 损失函数定义
对每张输入图像,记两个随机裁剪的global crops
为crops
组成集合
# teacher
输出的中心化与锐化
ViT
中没有使用BN
,使用的teacher
输出的中心化与锐化
中心化避免某个维度占据极端的影响,倾向于输出均匀分布,这有可能导致模型塌陷。
锐化可以增大维度之间的差异,因此同时使用中心化和锐化可以避免某个维度占据极端的影响的同时避免模型塌缩。
中心化是teacher
的输出减去center
,锐化就是对teacher
的输出使用温度系数
teacher_out = F.softmax((teacher_output - self.center) / temp, dim=-1)
teacher
输出的中心也使用了冲量更新:
# 模型总体结构及应用
backbone
+projection head
,backbone
输出的特征用于下游任务。
knn_classifier
使用的backbone
的cls_token
返回的shape
为(384,)的特征。
visualize_attention
使用的ViT
最后一层MSA
的output
的shape
为(n, L+1, L+1)
可视化只取output[:,0, 1:]
部分。
class Attention:
...
def forward(self, x):
B, N, C = x.shape
qkv = self.qkv(x).reshape(B, N, 3, self.num_heads, C // self.num_heads).permute(2, 0, 3, 1, 4)
q, k, v = qkv[0], qkv[1], qkv[2]
attn = (q @ k.transpose(-2, -1)) * self.scale
attn = attn.softmax(dim=-1)
attn = self.attn_drop(attn)
x = (attn @ v).transpose(1, 2).reshape(B, N, C)
x = self.proj(x)
x = self.proj_drop(x)
return x, attn